The Berlin Institute for Foundations of Learning and Data BIFOLD. New beacon of German AI research
Klaus-Robert Müller is one of the directors of the new Berlin Institute for Foundations of Learning and Data, BIFOLD for short. Read the exclusive interview here.
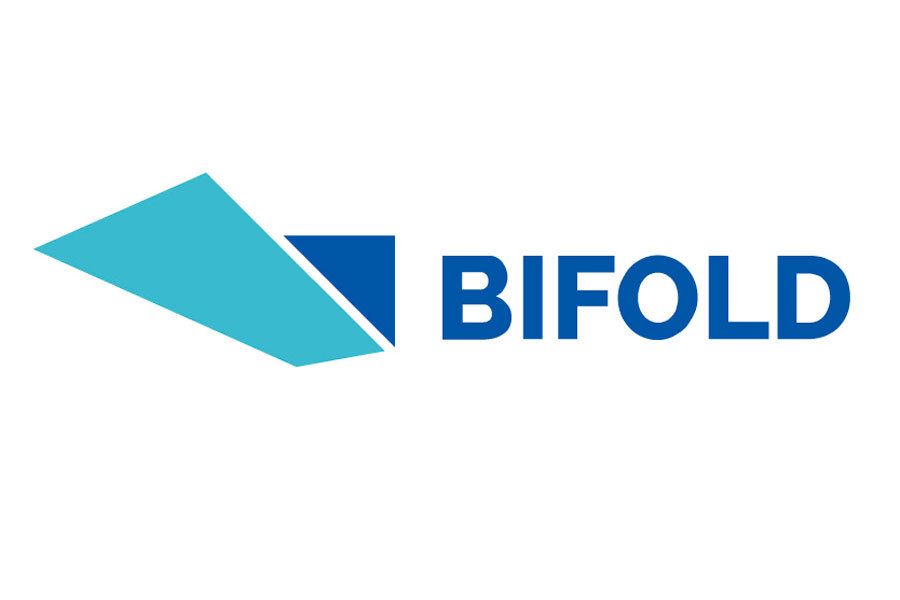
/BN, C. Duppé/ Klaus-Robert Müller has been working in the field of AI for more than 25 years. He is a member of the BCCN Berlin and was coordinator of the Bernstein Focus Neurotechnology until 2015. Since 2018, he is head of the Berlin Center for Machine Learning BZML, which is now merging with the Berlin Big Data Center BBDC to form BIFOLD, the Berlin Institute for Foundations of Learning and Data.
Bernstein Network (BN): Mr. Müller, the Berlin Institute for Foundations of Learning and Data, BIFOLD for short, is meant to become a beacon of German AI research on a global level. Where do you see the structural and substantial strengths of this new institute?
By merging two centers to form BIFOLD, we want to explore the technical foundations of AI in machine learning and database management. BIFOLD is intended to provide the opportunity to intensively research these technical core disciplines together and to interconnect them. Consequently, we aim at establishing interfaces to other scientific disciplines. On the one hand, the focus lies on managing the data itself and putting interesting questions to the data, for example how to deal with large data streams. At the same time, we seek to further develop of machine learning per se, especially core-based learning methods and deep learning.
In our institute we will therefore focus on three scientific fields: medicine, digital humanities and engineering.
This focus on basic research and the national strategic orientation shows a strong parallel to the Bernstein Network, which started in 2004 as a funding scheme of the Federal Ministry of Education and Research BMBF. Do you see similarities in the structural setup between the beginnings of the new AI centers, BIFOLD and the Bernstein Network?
As one of the co-founders of the Bernstein Center Berlin, I attended the meeting with the BMBF when the Bernstein idea was presented. First of all, it must be said that the Federal Government’s AI strategy is a lot bigger in terms of volume. The two are also very different regarding their scientific focus. The Bernstein Network focuses on computational neuroscience. The AI strategy of the Federal Government deals with AI in general, which is reflected in many areas, including, of course, applications in computational neuroscience.
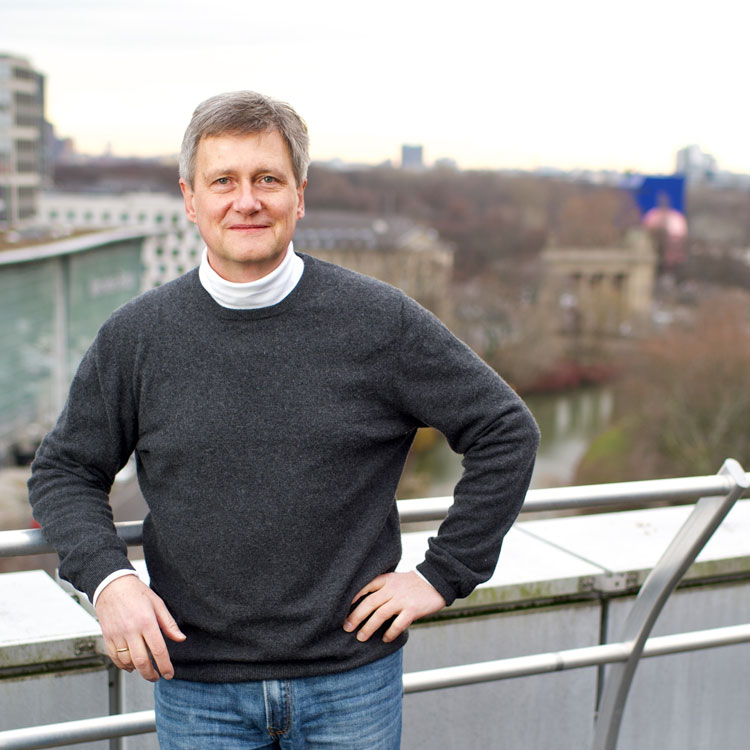
Klaus-Robert Müller, © TU Berlin/ Dominic Simon
Would the establishment of centers for machine learning as scientific hotspots reveal a structural parallel?
To a certain extent, yes. During the talks with the BMBF on the establishment of the centers, I have always pointed at the Bernstein Network and the Bernstein Centers as role models. The Bernstein Network is a success story one can learn from and in effect, one has. In the Bernstein Network, we have succeeded in creating an interdisciplinary educational concept that extends across the disciplines of cognitive science, neuroscience and mathematics to physics and computer science. The network has produced ‘multilingual’ researchers who are in great demand in today’s science. This is also one of the reasons for the boom in computational neuroscience and the great results that are rightly attributed to the Bernstein Network.
The AI centers are very similar, except for the fact that the disciplines are different. Before BIFOLD, machine learning and the big data disciplines existed parallel but they did not talk to each other. This will change with the new institute.
What exactly is the goal of BIFOLD in terms of applications?
In medicine, we deal with questions of genetics and pathology. In everyday clinical practice, the challenges lie, for instance, in intensive-care medicine, where robust algorithms for diagnosis and anomaly detection are lacking. This is what we are working on.
In quantum chemistry, we use machine learning to ‘solve’ the Schrödinger equation in a somewhat unconventional way. The breakthrough sounds relatively simple. If you want to solve the Schrödinger equation for one molecule, you can only do so approximately. To put it crudely, the approximation for solving the equation delighted a huge scientific field; it culminated in the Nobel Prize. It is a very active field – extremely relevant for physics, materials science and physical chemistry. The catch is the classical approximation (called density functional theory), which requires between 5 hours and 7 days of computing time per molecule. My recent work on machine learning for quantum chemistry has helped to replace this approximation – in other words, this complicated differential equation simulation step – with predictions of learning algorithms. In other words, it created a shortcut. This means that calculations can now be done within a millisecond; even predictions can be made with chemical accuracy for unknown molecules and materials.
What does BIFOLD do with regard to Digital Humanities?
The digital humanities are a new field at BZML, which we decided to focus on, since we saw that machine learning can be very beneficial in this area: basically, it is an approach that did not exist before, it can therefore produce new insights, such as a better understanding of the censorship of texts or intelligence about the propagation of images in the history of ideas.
Last but not least, we also engage in engineering, especially in (mobile) communication. In video streaming, for instance, it should be kept in mind that every second bit on the internet is encoded with the H264 standard; here we were able to achieve improvements with machine learning; they are already incorporated into the next generation of encoding standards. Even the new 5G standard cannot work without ML or Big Data. This is also what the new institute BIFOLD stands for: we work on applications of AI that affect us all.
Application orientation is a central theme at BIFOLD, because “Nothing is more practical than a good theory”. How can AI succeed in becoming a relevant economic factor?
AI has long been a huge economic factor. The big companies we all know and use on a daily basis are AI companies essentially. Larry Page once said Google is a machine learning company. In effect, AI has arrived in the economy a long time ago – maybe not in the consciousness of the German population to the extent that it could or should be – but fortunately a lot has happened in recent years.
This was one of the chief incentives for the AI strategy of the Federal Government. In the Federal Government’s last interim report, Europe was highlighted as a strong research location – with a number of patent applications ranked at the top even in comparison with China and the USA.
I believe that many of the things that are in the products of Internet companies emerge from Europe or even from Germany. We may not have founded Google, but this may not have been possible at the time. Casting a look into the future, we might ask ourselves which kind of boundary conditions are needed for such companies or such new economic sectors to emerge. Above all, it needs expertise and well-trained people. It also needs a new perception of AI. Companies must understand that they cannot continue the way they did. But don’t get me wrong: I believe that an incredible amount has happened in our country in recent years. Our government has also taken the first important steps. Certainly, more would be possible, still but I think in general we are on the right track.
Let’s talk briefly about explainable AI, which is one of your core objectives. Traceability and transparency are important to many people, especially when it comes to medical decisions. One of your chief concerns is data protection. Is there a German/European way of AI that approaches these issues differently than China or the USA?
Here I would like to carefully distinguish. Explainable AI is something that has indeed emerged in Germany – especially in Berlin. In 2010, I published the first paper of mine in this area. Back then we laid the foundations and today many people use our tools. Last March, some colleagues and I published a paper about the Clever Hans phenomenon: models can make good predictions, but for completely nonsensical and wrong reasons, i.e. the models use artefacts in the data for their predictions. This is highly dangerous. Therefore, transparency is very important so that engineers can improve their models and understand what is going wrong.
The question of ethics in AI is of course very important. As a matter of course we must consider ethical aspects of AI. However, one cannot exclusively deal with ethics in AI and leave the technical side to others, like the USA; one must consider them both together and educate people accordingly. In my opinion, one should not lapse into black and white painting and label the USA as the bad guys: I can remember very well that someone from Google management once proposed to work together with the United States Department of Defense – which led to so much resistance among the staff that this plan was shelved – a very impressive ethical statement.
At large. people must have easy-to-use technical tools at their disposal, which allow them to build fair, explainable and trustworthy AI. BIFOLD stands for that too.
Apart from ethics, the legal framework is also a decisive factor…
Yes, there is also a statement of the Leopoldina and the German Academy called Big Data and Privacy. I have led the project group on this. This paper covers the legal and technical aspects thereof. To cut a long story short: privacy is important to people in our society. In fact, we are increasingly losing it since we are giving away our data without reflection or because we do not create the framework to check on companies. This study examines what could be done technically and regulatively to regain a little more privacy.
At the same time, it is also important not to kill this wonderfully delicate plant of the many companies that are emerging in this area. This is certainly a challenging balancing act between raising the hurdles to regain privacy and new technical steps to explore new things. According to this, new rules have to be established by the Ministry of Justice, and we scientists have to react also. If everyone does their homework, in a few years’ time we will have systems that better protect our privacy.
BILFOLD is also supposed to create synergies through joining of research and business, especially through the training of a new generation of scientists who are not only active in research, but also beyond.
Berlin has quite along history of spin-offs and cooperations with industry. There are a lot of companies which cooperate with us, and BIFOLD will intensify this cooperation even more.
I believe we have been on a very good path for some time now. About 14-16 companies have emerged from my lab. These companies employ at least 500 people here in Berlin. But it is not only us. Many of my colleagues are at least as active when it comes to spin-offs. This is a sustainable economic factor.