The scientific case for brain simulations
HBP scientists argue for brain simulators as “mathematical observatories” for neuroscience
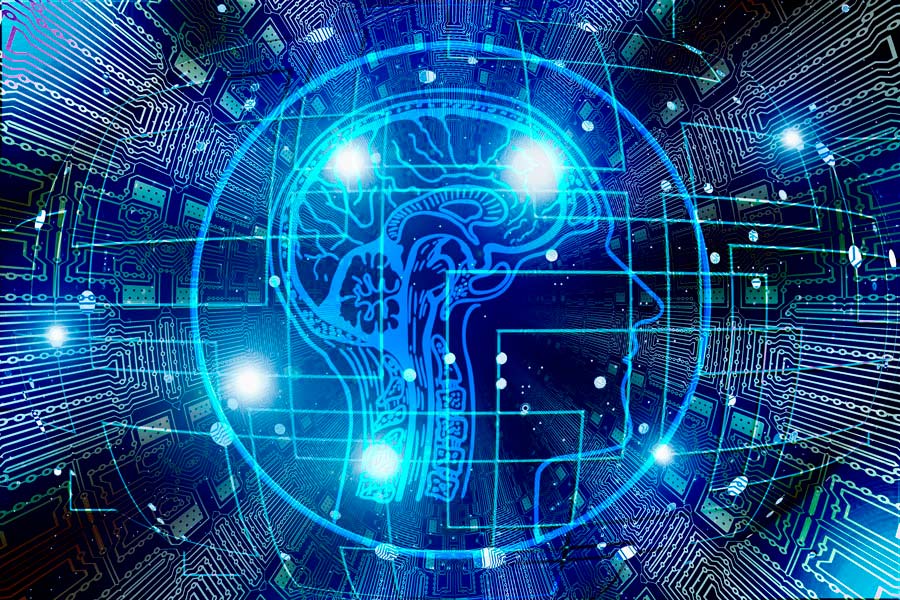
© Pixabay
Simulations of large-scale networks of neurons are a key element in the European Human Brain Project (HBP). In a new perspective article scientists from the HBP argue why such simulations are indispensable for bridging the scales between the neuron and system levels in the brain. The authors describe the need for open general-purpose simulation engines that can run a multitude of different candidate models of the brain at different levels of biological detail. Comparing predictions derived from such simulations with experimental data will allow systematic testing and refinement of models in a loop between computational and experimental neuroscience.
A wide variety of experimental techniques are used in neuroscience today to gain insight into neural function from measured brain signals. But to understand the complex nonlinear dynamics at play in the brain and to explain how the underlying activity gives rise to the signals, computational modeling is required. Simulations provide the crucial link between data generated by experimentalists and these models, a multi-author team, all affiliated with the European Flagship Human Brain Project, now writes in the new article “The scientific case for brain simulation”.
The basis for such simulations has been created on the HBP’s Brain Simulation Platform, a part of the projects Research Infrastructure for brain science that is openly accessible to the neuroscience community. The platform provides a set of continuously improved brain network simulators and has driven the construction of computational models and simulations at various scales, from single neurons, to large-scale brain-wide networks.
As the terms can easily be confused, the researchers emphasize that simulation and model are not identical. While mathematical models can embody many different hypotheses about how the brain works in equations and experimental parameters, “brain simulators can be viewed as ‘mathematical observatories’ to test various candidate hypotheses. A brain simulator is thus a tool, not a hypothesis, and can as such be likened to tools used to image brain structure or brain activity”, the scientists write. Simulation in this context means using sophisticated software tools to set complex models of the brain that represent large numbers of interconnected neurons into motion – and to observe what testable predictions emerge from them.
“The simulation does not represent the goal itself, but serves as a powerful new way for testing competing hypotheses about the brain”, explains Gaute Einevoll, Professor at the Norwegian University of Life Sciences (NMBU) and lead author of the paper. This then serves to enable a systematic “biological imitation game” where models that provide the best predictions of experimental data “win”.
To illustrate the point, Einevoll draws an analogy to the history of physics: “Our project can be compared to Isaac Newton’s development of a new branch in mathematics. Newton needed to develop a type of mathematics called calculus to check whether his proposed law of gravitation of how masses such as planets attract each other was correct or not. With it he could calculate the planetary paths in his model and verify that his theory was consistent with observations. With the simulation infrastructure we have developed, we can similarly test whether our candidate network models provide predictions that are consistent with brain measurements. This workflow will be important for further scientific progress, says Einevoll.
While the creation of detailed mathematical model has to integrate and generalize a wide range of data provided by experiments, the foundations of the network simulators are simpler and well established, the paper explains – “biophysical principles of how to model electrical activity in neurons and how neurons integrate synaptic inputs from other neurons and generate action potentials. These principles […] are the only hypotheses underlying the construction of brain network simulators. […] This is the reason why many models can be represented in the same simulator and why it is possible to develop generally applicable simulators for network neuroscience.”
“Over the past few years brain network simulators have matured tremendously and so have their scale and applications”, says co-author Markus Diesmann, a computational neuroscientist at Jülich Research Centre and one of the developers of the simulation engine NEST (Neural Simulation Tool). Within the HBP simulation engines like NEURON, Arbor, NEST or The Virtual Brain provide the backbone to address different levels of resolution and biological detail. Each offers specific advantages depending on the question.
“These well tested simulators play an important role in increasing the reproducibility of research by digitized workflows. Making progress here is crucial to be able to build on each other’s work”, says Sonja Grün, a data analytics expert at Jülich co-authoring the study. “What’s more is that it really creates a new culture of large-scale collaboration across experimental and theoretical neuroscience which we did not have until now”, Diesmann adds.
This collaborative approach, which is well-established in disciplines like physics or astronomy, is a crucial step in approaching the staggering complexity of the brain, the scientists emphasize in their paper:
“Newton said that he had seen further than others because he was ‘standing on the shoulders of giants.’ Likewise, we argue that we need to find a way to stand on the shoulders of each other’s mathematical models to hope to gain a detailed understanding of the functioning of brain networks.”
Text in part adapted from HBP partner NMBU
Original publication
https://www.cell.com/neuron/fulltext/S0896-6273(19)30290-9